What is Inter Rater Reliability (IRR)?
Inter-Rater Reliability (IRR) is a statistical concept used in healthcare data collection and analysis. In the context of clinical data abstraction, inter-rater reliability refers to the degree of agreement between two or more abstractors when they independently review and extract data from clinical records or other medical sources. IRR measures the extent to which the data collected by different individuals is consistent, ensuring the abstraction output is reliable, reproducible, and not overly influenced by the subjectivity or bias of individual abstractors. Beyond abstractor agreement, IRR can also help assess your team’s understanding and application of the specifications, as well as identify knowledge gaps that should be targeted for focused review and staff education.
Why Should Inter Rater Reliability Matter to You?
Abstracting data from patients’ medical records is a widespread practice and a primary method for capturing key clinical data elements. The resulting information is analyzed, interpreted, and used for vitally important work like clinical decision-making and treatment plans, performance measurement and peer comparisons, calculating incidence, prevalence, cost, and more. Given the growing demand for data by expanding audiences with varied purposes, data integrity has never been more paramount.
High IRR is critical as it ensures that data gathered from clinical sources can be trusted, whether used for research, quality assurance, or policy formulation. External entities and accrediting bodies like the Centers for Medicare & Medicaid Services (CMS) evaluate the data submitted to their warehouses and have differing processes for validating the information before its publicly reported. As an industry leader in data abstraction for over a decade, ADN has the experience, tools, and processes to maintain accuracy at much higher rates than the acceptable minimum of accrediting bodies. ADN’s team upholds an accuracy rate of 95% or higher across all clients, all abstractors, and all measures and registries. Only with dependable, high-fidelity data can your organization confidently utilize abstraction insights to increase compliance with evolving standards and continuously improve the overall quality and safety of care.
“We make significant investments in inter-rater reliability to ensure the integrity of our data abstractions and to continuously monitor staff competency. While it is resource and time-intensive to sustain the necessary redundancies, IRR has a proven rate of return. Accurate results are the cornerstone of our trusted relationships with our staff and partners, and the value extends to our client’s senior leaders, physicians and other clinicians who use abstracted registry data to drive care decisions.”
–Dalana Pittman, ADN’s Director of Data Abstraction Services
How To Assess Inter Rater Reliability
The most straightforward IRR formula is calculated as a percentage of agreement between the original abstractor and the reviewer. Ideally, the reviewer is a trained, experienced abstractor who independently re-abstracts a sample of cases that were already completed by the original abstractor. Then, their data element responses are directly compared to examine inter rater agreement and isolate mismatches.
The two most common methods for evaluating inter-rater reliability are the Data Element Agreement Rate (DEAR) and the Category Assignment Agreement Rate (CAAR). For how to calculate these agreement rates, read “Inter-Rater Reliability: What It Is, How to Do It, and Why Your Hospital’s Bottom Line Is at Risk Without It.”
- DEAR is a one-to-one comparison of consensus between the original abstractor and the reviewer’s findings at the data element level, including all clinical and demographic elements. DEAR results should be analyzed for trends among mismatches within a specific data element or for a particular abstractor to determine if an additional, focused review is needed to ensure accuracy across all potentially affected charts.
- CAAR is a one-to-one comparison of agreement between the original abstractor and the reviewer’s record-level results using Measure Category Assignments (MCAs) and should be used to identify the overall impact of data element mismatches on measure outcomes.CAAR scores are most applicable to Core Measure populations and are a predictor of CMS Validation results.
An easy and reliable way to compare data element responses and calculate the agreement between raters is to use a prebuilt IRR Template, which is available for free in ADN’s Inter-Rater Reliability Toolkit (see screenshots below). However, it’s important to keep in mind that if you’re looking to stand up a new IRR program or expand your existing one, that’s more time your already swamped staff will be spending on collecting the data rather than leveraging it to drive change. The whole value premise of outsourcing data abstraction (and the accompanying IRR work) to a partner like ADN is to stop spending precious in-house resources on data collection and instead reallocate those employee hours to data analysis and performance improvement.
It’s a zero-sum game: Any time spent collecting data is time not spent improving care. Economists call it an “opportunity cost.” We call it a distraction from your core competency of elevating care quality.
If you plan to use this template to enhance your internal processes, here’s an example of what an abstraction mismatch looks like using ADN’s IRR Sepsis Calculation Template (which functions in Excel or Google Sheets).
DOWNLOAD ADN’S IRR TOOLKIT
Best Practices to Achieve High IRR for Clinical Data Abstraction
As an industry leader in clinical data abstraction who’s worked with these types of data sets since the inception of Core Measures, we’ve learned several things over the years. Here are a few best practices your organization can follow.
1. Establish an IRR Program and Policy
In addition to traditional policy components (e.g., purpose, definitions, roles/responsibilities), a comprehensive IRR Program and Policy should address the following:
- Cross-Training & Buddy System
- IRR Sampling Methodology
- Schedule & Timeliness of Results
- Ongoing and Focused Assessments
- Agreement Rate Calculations & Acceptable Thresholds
- Abstractor Feedback Loop
- Education & Remediation Plans
Once all the key components of the program are finalized, the next step is to create a detailed policy that outlines the Standard Operating Procedures. Whether you already have a policy in place or are just getting started, consider using the IRR Policy Template included in ADN’s IRR Toolkit as a springboard for your new or improved program.

2. Go Beyond Scheduled Reviews. Conduct IRR on “Trigger Events.”
Certain events or “triggers” introduce a higher-than-average likelihood for an error to occur. Having been a clinical data abstraction outsourcing provider for more than 13 years, we’ve compiled a list of events that prompt IRR. Incorporating the following into your IRR processes will allow for earlier detection and quality control, thereby increasing the accuracy and integrity of the data your organization uses to make decisions.
- Version updates
- New hires or assignment changess
- New physicians and/or documentation practices
- New measures or populations
- Technology changes (e.g., EHR)
- Performance evaluations
Even the best-laid plans can go awry without a standardized process and set of tools. As an abstraction vendor since 2010, ADN has refined and optimized our processes to be as near to an exact science as you can get. And as part of our mission, we wanted to make some of those tools available for free.
But we still can’t stress enough that organizations recognized as leaders in the healthcare industry are choosing to proactively outsource processes like data abstraction so they can achieve laser focus on their core competency – improving care quality.
We highly recommend a brief 30-minute conversation with our team to get a pricing proposal so you can make an informed decision on how to best allocate your scarce internal resources. Abstraction outsourcing is much more affordable than you might think. Click here to grab 30 minutes on our team’s calendar.
Download ADN’s IRR Toolkit here.
4. Use IRR to Evaluate Competencies & Tailor Education Plans
IRR assessments can be an effective way to test abstractor competency and serve as the bedrock of your education strategy. In addition to calculating chart-level agreement rates, consider also tracking agreement at the data element level to pinpoint opportunities and tailor individual training plans. For your focused IRR assessments, we suggest selecting data elements that have consequential impacts on the data or outcomes, such as fields affecting risk adjustment (e.g., STS-ACS > Beta Blocker Within 24 Hours, Discharge Medications, Post-Op Complications) and/or those that significantly influence the skip logic cascade (e.g., Sepsis > Transfer From Another Hospital, Comfort Measures Only, Severe Sepsis Present, Septic Shock Present).
Closing the feedback loop is another critical step in the process. Findings from IRR assessments should be shared with all relevant abstractors to foster shared learning, as some data elements are germane across multiple measure sets/registries (e.g., ED Admit Time, Arrival Date and Time, Discharge Date and Time). Not only is communication key, but ADN recommends taking it one step further by updating your internal documentation tendency blueprints to reflect lessons learned. This approach to IRR can help to more tightly align abstractions across your facility and/or enterprise so that individuals are using the same source data and fields to abstract cases.
As with any new or revitalized program, it is important to communicate openly with the abstraction team to obtain buy-in well in advance of the launch date. We recommend emphasizing that the purpose of an IRR program is to increase overall accuracy and bolster organizational confidence in data-driven decisions. For optimal success, it is imperative that the abstraction staff understands their roles and responsibilities within the program, and moreover trusts that any IRR findings will be used for learning and not disciplinary purposes. With that in mind, abstractors can still be held accountable for meeting and sustaining acceptable DEAR and CAAR rates; so ADN recommends aligning your abstractor job descriptions and annual goal-setting with these established thresholds.
Challenges in Achieving High IRR
While the importance of Inter-Rater Reliability in healthcare is undeniable, achieving a high level of consistency and agreement among data abstractors is not without its challenges. These range from the inherent complexities of clinical data to the evolving landscape of medical documentation and technology. Addressing these hurdles is crucial, as robust IRR ensures that healthcare decisions are based on accurate, reliable data. In this section, we’ll delve into the multifaceted obstacles that healthcare facilities often face in their quest to achieve and maintain high inter rater reliability.

1. Training and Skill Variability
Diverse Backgrounds: Abstractors hail from a myriad of educational and professional backgrounds, such as nursing, health informatics, or medical coding. This diversity, while fostering a rich pool of expertise, can sometimes lead to different interpretations of the same data.
Training Consistency: In organizations with numerous departments or systems with multiple facilities, ensuring that every abstractor receives the same caliber of training is a logistical challenge. High turnover rates exacerbate this issue, as new hires need to be brought up to speed swiftly, risking potential oversights or inconsistencies in training delivery.
Varied Interpretation of Ever-Changing Standards: Abstraction guidelines are constantly receiving updates from measure and registry stewards like CMS, AHA, NCDR, and the ACC. When new standards are introduced, there’s often a transition period where guidelines might be open to interpretation. During this phase, abstractors are still facing a learning curve that can lead to inconsistencies within the data set.
2. Complexity of Clinical Data
Vague or Ambiguous Data: Medical records can sometimes contain ambiguous or incomplete information, especially unstructured data like clinical notes with complexities like acronyms, abbreviations, and spelling/grammatical errors. Lack of clarity can lead to multiple valid interpretations, making it difficult to achieve consistent data abstraction.
Evolving Medical Practices: Medicine is a rapidly advancing field with new research and evolving evidence-based practices that lead to frequent updates in data collection guidelines. Abstractors must remain current regarding these shifts to ensure their interpretations align with the most current specification versions.
3. Documentation Practices
Physician Variability: Based on their training and experience, physicians often have unique documentation styles and preferences. This can pose challenges for abstractors who must interpret and abstract data from multiple physicians with differing approaches to similar clinical scenarios. Additionally, variation in how and where physicians document within the EHR can cause missed or misinterpreted information.
Updates in Documentation Standards: Medical documentation standards such as ICD or CPT codes undergo periodic revisions. Clinical data abstractors should understand the impact of these coding changes on their patient populations (e.g., new inclusion/exclusion criteria).
4. Subjectivity in Data Interpretation
Bias: Everyone has inherent biases based on their experiences, beliefs, and training. These biases, even if subtle, can influence how an abstractor interprets ambiguous or subjective data.
External Influences: Factors like workload, stress, or even the time of day can subtly influence how data is interpreted. For instance, an abstractor working after a long shift might interpret data differently than when they’re fresh.
5. Lack of Regular Feedback
Delayed Corrections: Without regular feedback, abstractors might continue making the same interpretation errors. Over time, these uncorrected errors can compromise the integrity of the entire data set.
Missed Learning Opportunities: Regular feedback sessions can also serve as mini-training sessions, reinforcing the correct interpretation practices. IRR shouldn’t just be about isolating and rectifying mismatches; it’s a powerful learning tool that can highlight knowledge gaps, reinforce best practices, and serve as the cornerstone of your abstractor education strategy.
6. Resource Limitations
Time Constraints: In fast-paced environments and high-volume facilities, abstractors are under significant pressure for timely turnarounds. Unfortunately, this can lead them to rush through data interpretations and cause potential errors.
Lack of Dedicated IRR Teams: Not every facility has the resources to maintain a dedicated IRR team. Thus, the responsibility and burden often falls on a few individuals, making it challenging to complete IRR in a timely manner. Without sufficient sampling and meaningful feedback sessions between abstractors, errors can go undetected and negatively impact the data’s overall reliability.
Elevate Your IRR with ADN
IRR forms the foundation of accurate healthcare data interpretation. By implementing best practices and continuous improvement, you can achieve high inter rater reliability and dramatically advance patient outcomes. Recognizing the importance of establishing and maintaining a strong data collection process is only the first step. Fully and confidently leveraging the power and value of your abstracted data will involve investing time and expertise in an IRR program. But fret not if you lack the resources or time to fully implement this toolkit. We can handle the heavy lifting by helping to organize and strengthen your collection and validation processes, while your team focuses on interpreting the data and applying lessons learned to provide better care. ADN can help you chart a course to a future of reliable data—here’s how.
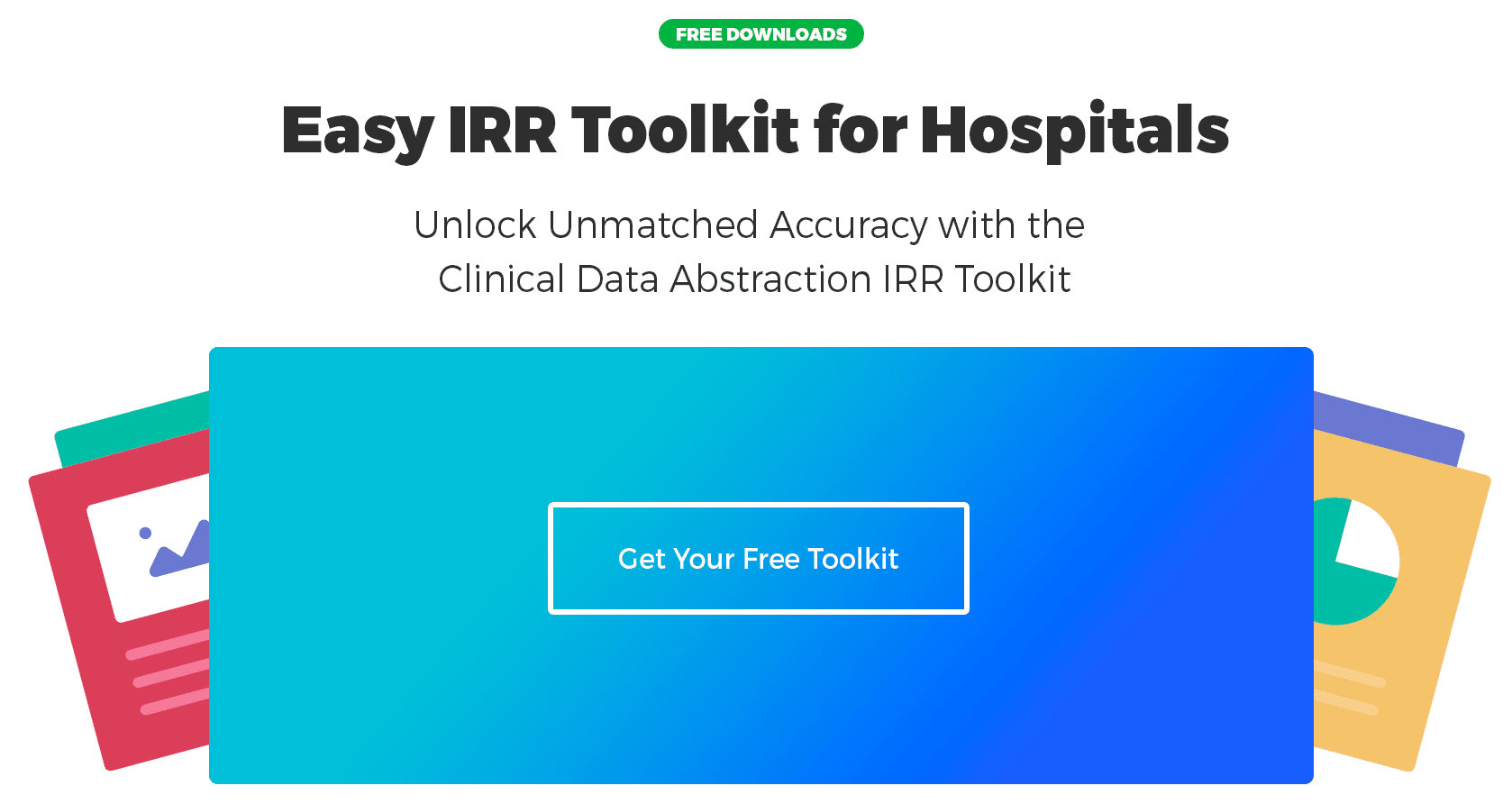